Last updated on June 28th, 2023 at 02:43 pm
Mention “AI music” and most people seem to think of AI-generated music. In other words, they picture a robot, machine or application composing, creating and possibly performing music by itself; essentially what musicians already do very well. First, let’s address every industry professional’s worst Terminator-induced fears (should they have any): AI will never replace musicians.
Even if music composed and generated by AI is currently riding a rising wave of hype (include link to previous article), we’re far from a scenario where humans aren’t in the mix. The perception around AI infiltrating the industry comes from a lack of attention towards what AI can actually do for music professionals. That’s why it’s important to cut through the noise and discuss different use cases possible right now.
Let’s look at three ways to use AI in the music industry and why they should be embraced.
AI-based Music Generation
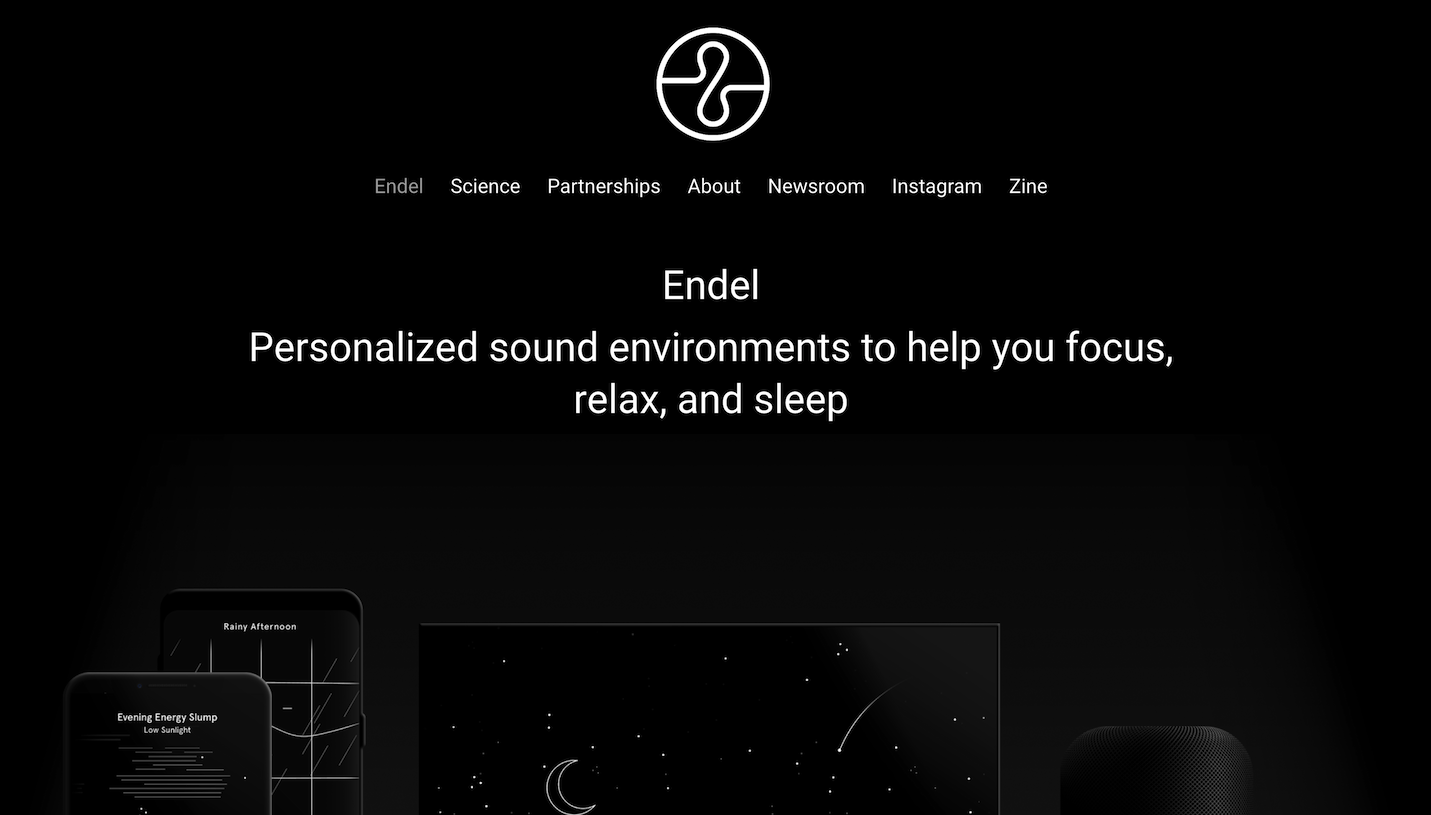
The most popular application of AI in music is in the field of AI-generated music. You might’ve have heard about AIVA and Endel (which sound like the names of a pair of northern European fairy-tale characters). AIVA, the first AI to be recognized as a composer by the music world, writes entirely original compositions. Last year, Endel, an AI that creates ambient music, signed a distribution deal with Warner Music. Both these projects signal a shift towards AI music becoming mainstream.
Generative music systems are built on machine learning algorithms and data. The more data you have, the more examples an algorithm can learn from, leading to better results after it’s completed the learning process – this is known in AI-circles as ‘training’. Although AI-generation doesn’t deliver supremely high quality yet, some of AIVA’s supposed self-made compositions stack up well compared against modern composers.
If anything, it’s the chance for co-creation that excites today’s musicians. Contemporary artists like Taryn Southern and Holly Herndon use AI technology to varying degrees, with drastically different results. Southern’s pop-ready album, I AM AI, released in 2018. It was produced with the help of AI music-generating tools such as IBM’s Watson and Google’s Magenta.
Magenta is included in the latest Ableton Live release, a widely-used piece of music production software. As more artists begin to play with AI-music tools like these, the technology becomes an increasingly valuable creative partner.
AI-based Music Editing
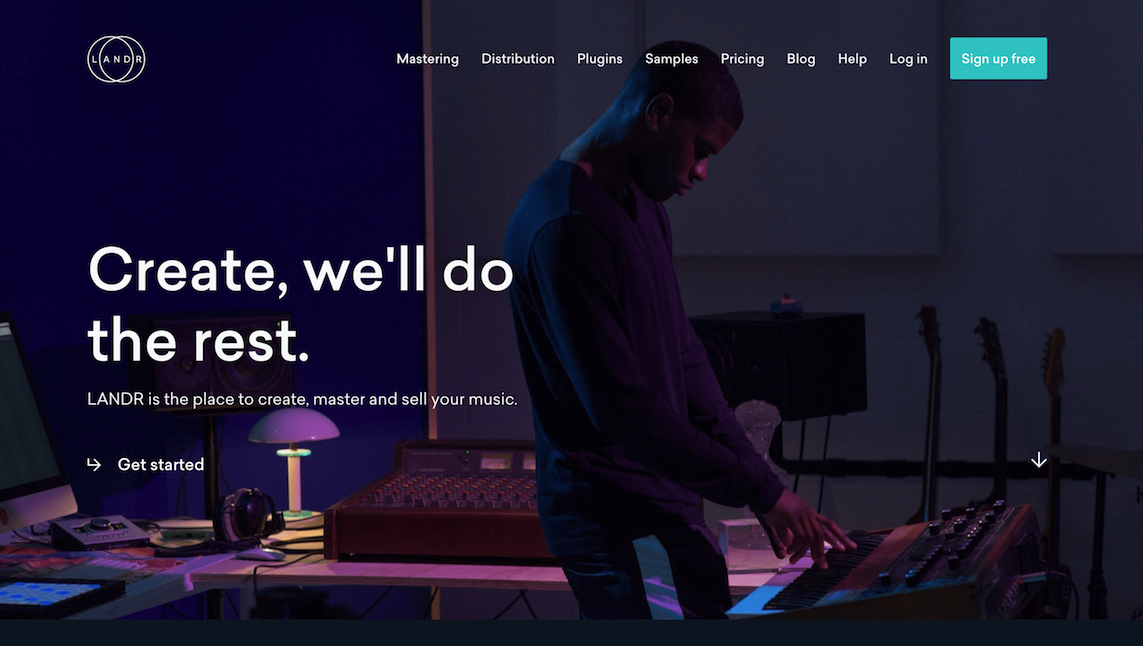
Before the music arrives for your listening pleasure, it undergoes a lengthy editing process. This includes everything from mixing the stems – the different grouped elements of a song, like vocals and guitars – to mastering the finished mixdown (the rendered audio file of the song made by the sound engineer after they’ve tweaked it to their liking).
This whole song-editing journey is filled with many hours of attentive listening and considered action. Because of the amount of choices involved, having an AI to assist in making technical suggestions can speed things up. Equalization is a crucial editing step, which is as much technical as it is artistic. This refers to an audio engineer balancing out the specific frequencies of a track’s sounds, so they complement rather than conflict with each other. Using an AI to perform these basic EQ functions can provide an alternative starting point for the engineer.
Another example of fine-tuning music for consumption is the mastering process. Because published music must stick to strict formatting to for radio and TV, or film, it needs to be mastered. This final step before release usually requires a mastering engineer. They basically make the mix sound as good as possible, so it’s ready for playback on any platform.
Some of the technical changes mastering engineers make are universal. For example, they need to make every mixdown louder to match the standard of music that’s out there; or even to match the other songs on an album. Using universal techniques means AI can help, because you’ve got practices it can learn from. These practices can then be automatically applied and tailored to the song.
Companies like LANDR and Izotope are already on board. LANDR offers an AI-powered mastering service that caters to a variety of styles, while Izotope developed a plugin that includes a “mastering assistant“. Once again, AI can act as a useful sidekick for those spending hours in the editing process.
AI-based Music Analysis
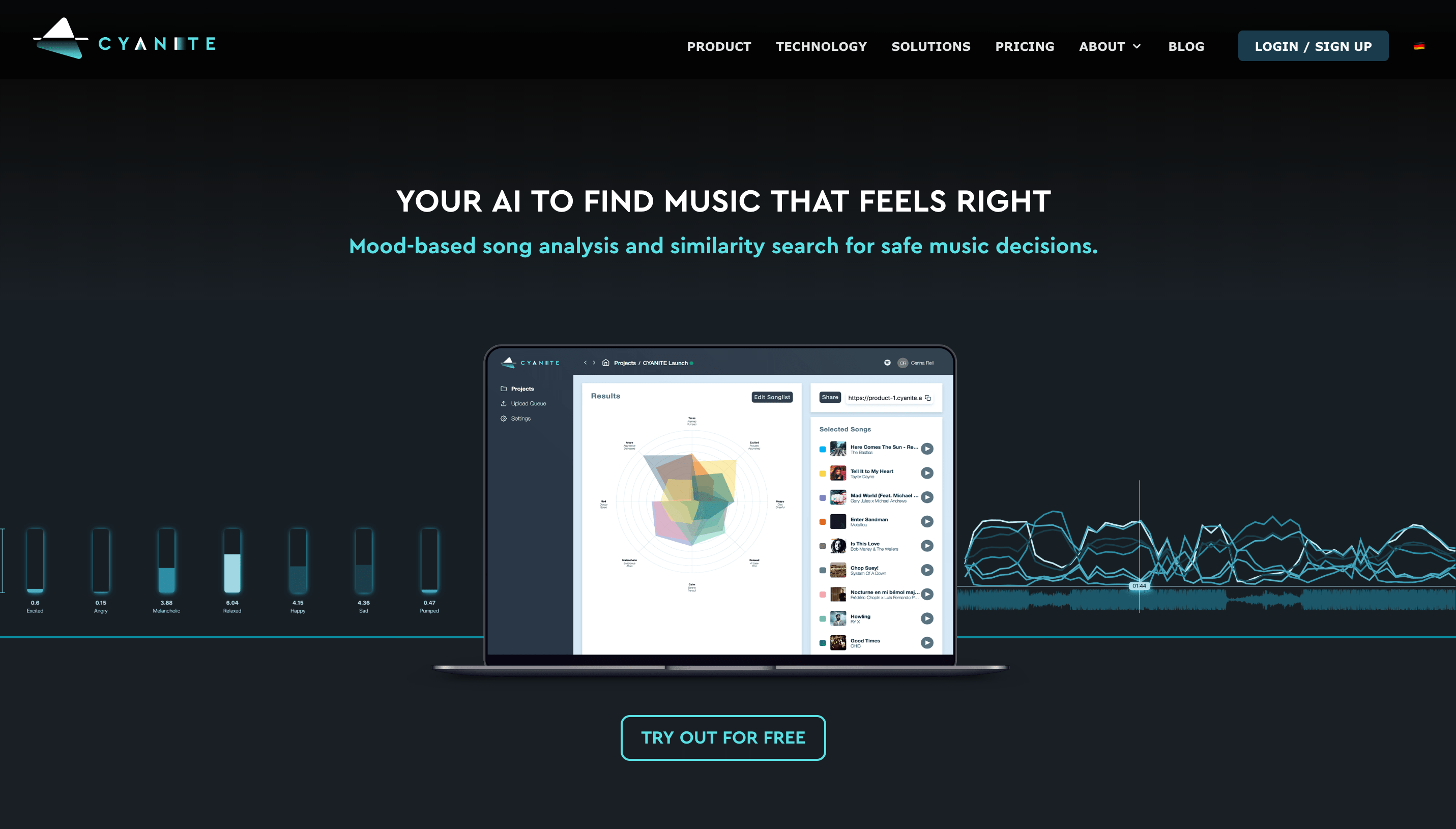
Analysis is what happens when you break something down into smaller parts. In AI music terms, analysis is the process of breaking down a song into parts. Let’s say you’ve got a library full of songs and you’d like to identify all the exciting orchestral music (maybe you’re making a trailer for the next Avengers-themed Marvel movie). Through AI, analysis can be performed to highlight the most relevant music for your trailer based on your selected criteria (exciting; orchestral).
There are two types of analysis that make this magic possible: symbolic analysis and audio analysis. While symbolic analysis gathers musical information about a song from the score – including the rhythm, harmony and chord progressions, for example – audio or waveform analysis considers the entire song. This means understanding what’s unique about the fully-rendered wave (like those you see when you hit play on SoundCloud) and comparing it against other waves. Audio analysis enables the discovery of songs based on genre, timbre or emotion.
Both symbolic and audio analysis use feature extraction. Simply put, this is when you pull numbers out of a dataset. The better your data – meaning quality, well-organized and clearly tagged – the easier it is to pick up on ‘features’ of your music. These could be ‘low-level’ features like loudness, how much bass is present or the type of rhythms common in a genre. Or they could be ‘high level’ features, referring more broadly to the artist’s style, based on lyrics and the combination of musical elements at play.
AI-based music analysis makes it easier to understand what’s unique about a group of songs. If your algorithm learns the rhythms unique to Drum and Bass music, it can discover those songs by genre. And if it learns how to spot the features that make a song “happy” or “sad”, then you can search by emotion or mood. This allows for better sorting, and finding exactly what you pictured. Better sorting means faster, more reliable retrieval of the music you need, making you project process more efficient and fun.
With Cyanite we offer music analysis services via an API solution to tackle large music databases or the ready-to-use web app CYANITE. Create a free account to test AI-based tagging and music recommendations.