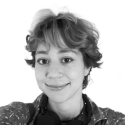
Eylül
Data Scientist at Cyanite
This is part 1 of 2. To dive deeper into the data we analyzed, click here to check out part 2.
Gender Bias in AI Music: An Introduction
Gender Bias in AI Music Search is often overlooked. With the upcoming release of Cyanite 2.0, we aim to address this issue by evaluating gender representation in AI music algorithms, specifically comparing male and female vocal representation across both our current and updated models.
Finding music used to be straightforward: you’d search by artist name or song title. But as music catalogs have grown, professionals in the industry need smarter ways to navigate vast libraries. That’s where Cyanite’s Similarity Search comes in, offering an intuitive way to discover music using reference tracks.Â
In our evaluation, we do not want to focus solely on perceived similarity but also on the potential gender bias of our algorithm. In other words, we want to ensure that our models not only meet qualitative standards but are also fair—especially when it comes to gender representation.Â
In this article, we evaluate both our currently deployed algorithms Cyanite 1.0 and Cyanite 2.0 to see how they perform in representing artists of different genders, using a method called propensity score estimation.
Cyanite 2.0 – scheduled for Nov 1st, 2024, will cover an updated version of Cyanite’s Similarity and Free Text Search, scoring higher in blind tests measuring the similarity of recommended tracks to the reference track.
Why Gender Bias and Representation Matters in Music AI
In machine learning (ML), algorithmic fairness ensures automated systems aren’t biased against specific groups, such as by gender or race. For music, this means that AI music search should equally represent both male and female artists when suggesting similar tracks.
An audio search algorithm can sometimes exhibit gender bias as an outcome of a Similarity Search. For instance, if an ML model is trained predominantly on audio tracks with male vocals, it may be more likely to suggest audio tracks that align with traditional male-dominated artistic styles and themes. This can result in the underrepresentation of female artists and their perspectives.
The Social Context Behind Artist Representation
Music doesn’t exist in a vacuum. Just as societal biases influence various industries, they also shape music genres and instrumentation. Certain instruments—like the flute, violin, and clarinet—are more often associated with female artists, while the guitar, drums, and trumpet tend to be dominated by male performers. These associations can extend to entire genres, like country music, where studies have shown a significant gender bias with a decline in female artist representation on radio stations over the past two decades.Â
What this means for AI Music Search models is that if they aren’t built to account for these gendered trends, they may reinforce existing gender- and other biases, skewing the representation of female artists.
How We Measure Fairness in Similarity Search
At Cyanite, we’ve worked to make sure our Similarity Search algorithms reflect the diversity of artists and their music. To do this, we regularly audit and update our models to ensure they represent a balanced range of artistic expressions, regardless of gender.
But how do we measure whether our models are fair? That’s where propensity score estimation comes into play.
What Are Propensity Scores?
In simple terms, propensity scores measure the likelihood of a track having certain features—like specific genres or instruments—that could influence whether male or female artists are suggested by the AI. These scores help us analyze whether our models are skewed toward one gender when recommending music.
By applying propensity scores, we can see how well Cyanite’s algorithms handle gender bias. For example, if rock music and guitar instrumentation are more likely to be associated with male artists, we want to ensure that our AI still fairly recommends tracks with female vocals in those cases.
Picture 1: We aim for gender parity in each bin, meaning the percentage of tracks with female vocals should be approximately 50%. The closer we are to that horizontal purple dashed line, the better our algorithm performs in terms of gender fairness.
Comparing Cyanite 1.0 and Cyanite 2.0
To evaluate our algorithms, we created a baseline model that predicts the likelihood of a track featuring female vocals, relying solely on genre and instrumentation data. This gave us a reference point to compare with Cyanite 1.0 and Cyanite 2.0.
Take a blues track featuring a piano. Our baseline model would calculate the probability of female vocals based only on these two features. However, this model struggled with fair gender representation, particularly for female artists in genres and instruments dominated by male performers. The lack of diverse gender representation in our test dataset for certain genres and instruments made it difficult for the baseline model to account for societal biases that correlate with these features.
The Results
The baseline model significantly underestimated the likelihood of female vocals in tracks with traditionally male-associated characteristics, like rock music or guitar instrumentation. This shows the limitations of a model that only considers genre and instrumentation, as it lacks the capacity to handle high-dimensional data, where multiple layers of musical features influence the outcome.
In contrast, Cyanite’s algorithms utilize rich, multidimensional embeddings to make more meaningful connections between tracks, going beyond simple genre and instrumentation pairings. This allows our models to provide more nuanced and accurate predictions.
Despite its limitations, the baseline model was useful for generating a balanced test dataset. By calculating likelihood scores, we paired male vocal tracks with female vocal tracks that had similar characteristics using a nearest-neighbour approach. This helped eliminate outliers, such as male vocal tracks without clear female counterparts and resulted in a balanced dataset of 2,503 tracks, each with both male and female vocal representations.
When we grouped tracks into bins based on the likelihood of female vocals, our goal was a near-equal presence of female vocals across all bins, with 50% representing the ideal gender balance. We conducted this analysis for both Cyanite 1.0 and Cyanite 2.0.
The results were clear: Cyanite 2.0 produced the fairest and most accurate representation of both male and female artists. Unlike the baseline model and Cyanite 1.0, which showed fluctuations and sharp declines in female vocal predictions, Cyanite 2.0 consistently maintained balanced gender representation across all probability ranges.
To see more explanation on how propensity scores can help aid gender bias in AI music and balance the gender gap, check out part 2 of this article.
Conclusion: A Step Towards Fairer Music Discovery
Cyanite’s Similarity Search has applications beyond ensuring gender fairness. It helps professionals to:
- Use reference tracks to find similar tracks in their catalogs.
- Curate and optimize playlists based on similarity results.
- Increase the overall discoverability of a catalog.
Our comparative evaluation of artist gender representation highlights the importance of algorithmic fairness in music AI. With Cyanite 2.0, we’ve made significant strides in delivering a balanced representation of male and female vocals, making it a powerful tool for fair music discovery.
However, it’s crucial to remember that societal biases—like those seen in genres and instrumentation—don’t disappear overnight. These trends influence the data that AI music search models and genAI models are trained on, and we must remain vigilant to prevent them from reinforcing existing inequalities.
Ultimately, providing fair and unbiased recommendations isn’t just about gender—it’s about ensuring that all artists are represented equally, allowing catalog owners and music professionals to explore the full spectrum of musical talent. At Cyanite, we’re committed to refining our models to promote diversity and inclusion in music discovery. By continuously improving our algorithms and understanding the societal factors at play, we aim to create a more inclusive music industry—one that celebrates all artists equally.
If you’re interested in using Cyanite’s AI to find similar songs or learn more about our technology, feel free to reach out via mail@cyanite.ai.
You can also try our free web app to analyze music and experiment with similarity searches without needing any coding skills.