Last updated on June 28th, 2023 at 02:38 pm
Artificial intelligence is an innovative technology. Pair it with a music library, and you get innovative results. That’s largely thanks to an AI music approach called MIR – Music Information Retrieval. What’s great about approaches like MIR is that they give you the power to find the exact song you’re looking for.
There are many ways you can integrate AI into your catalogue. We’ve identified three that are both easy on the eyes and rich in information. Let’s dive into the three most effective tools for presenting AI-generated results in your library or online platform.
Mood/Colour Visualisation
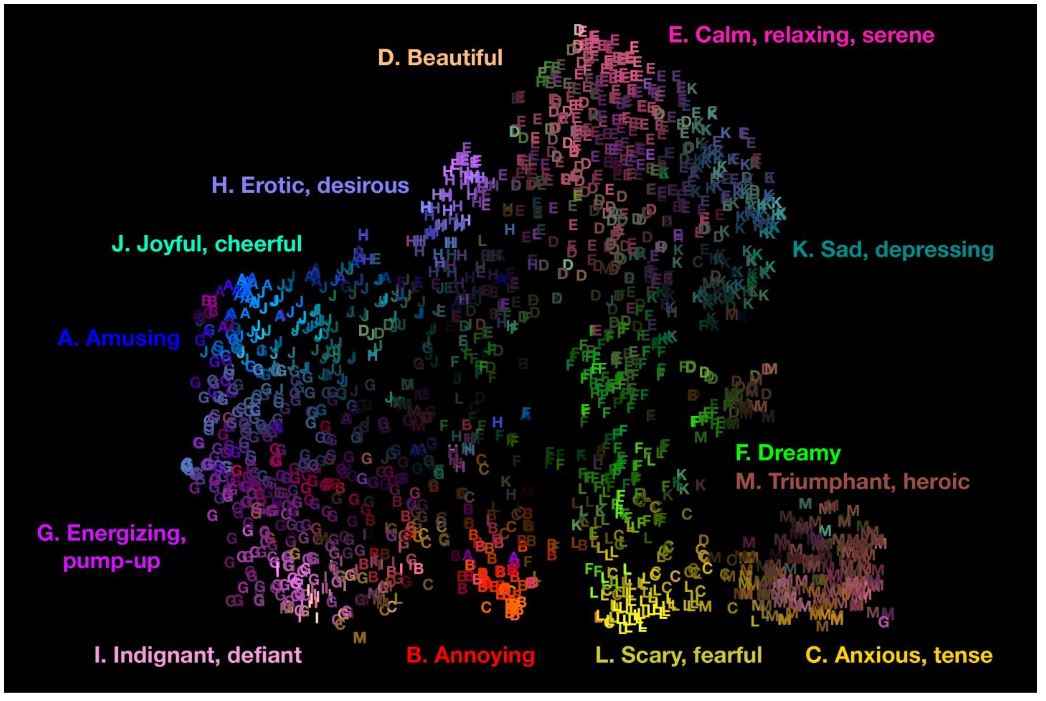
This map from UC Berkeley matches colors to emotional responses from music
The world is a colourful place. You’ll find different shades and hues everywhere you look. And that’s great, because colours are intuitive to understand. We’re used to making sense of the world through them. Traffic lights and street signs work that way. So does fashion.
There’s also a clear connection between psychology and colours. Whether natural or learned behaviour, we attach moods to colours. If someone mentions sad, what’s the first colour you think of? What about happy, angry or excited? You probably guessed right, and you didn’t have to think about it for very long either.
Because music is an art form that’s all about mood and emotion, it makes sense to match songs to colours. That’s exactly what the University of California, Berkeley did. They surveyed 2500 people from the United States and China. The aim was to test their emotional responses to thousands of songs from genres such as classical, rock, jazz, folk, experimental and heavy metal. Researchers then determined 13 feelings to map out the subjective experience of music: “Amusement, joy, eroticism, beauty, relaxation, sadness, dreaminess, triumph, anxiety, scariness, annoyance, defiance, and feeling pumped up.”
Colour-based visualisation is great because it’s easy to navigate and provides analysis-driven results. Songs are grouped by emotion, and you see exactly how many fill each group. You also get a thorough first impression about the general structure of the song.
If you want to see (and hear) for yourself, check out this interactive audio map created from the data. Listeners can switch tunes to try out specific moods, and see how much of an emotion is present in a song. (50% romantic, 25% dreamy and 4% nauseating sounds like a rock-solid combination.)
Song Maps
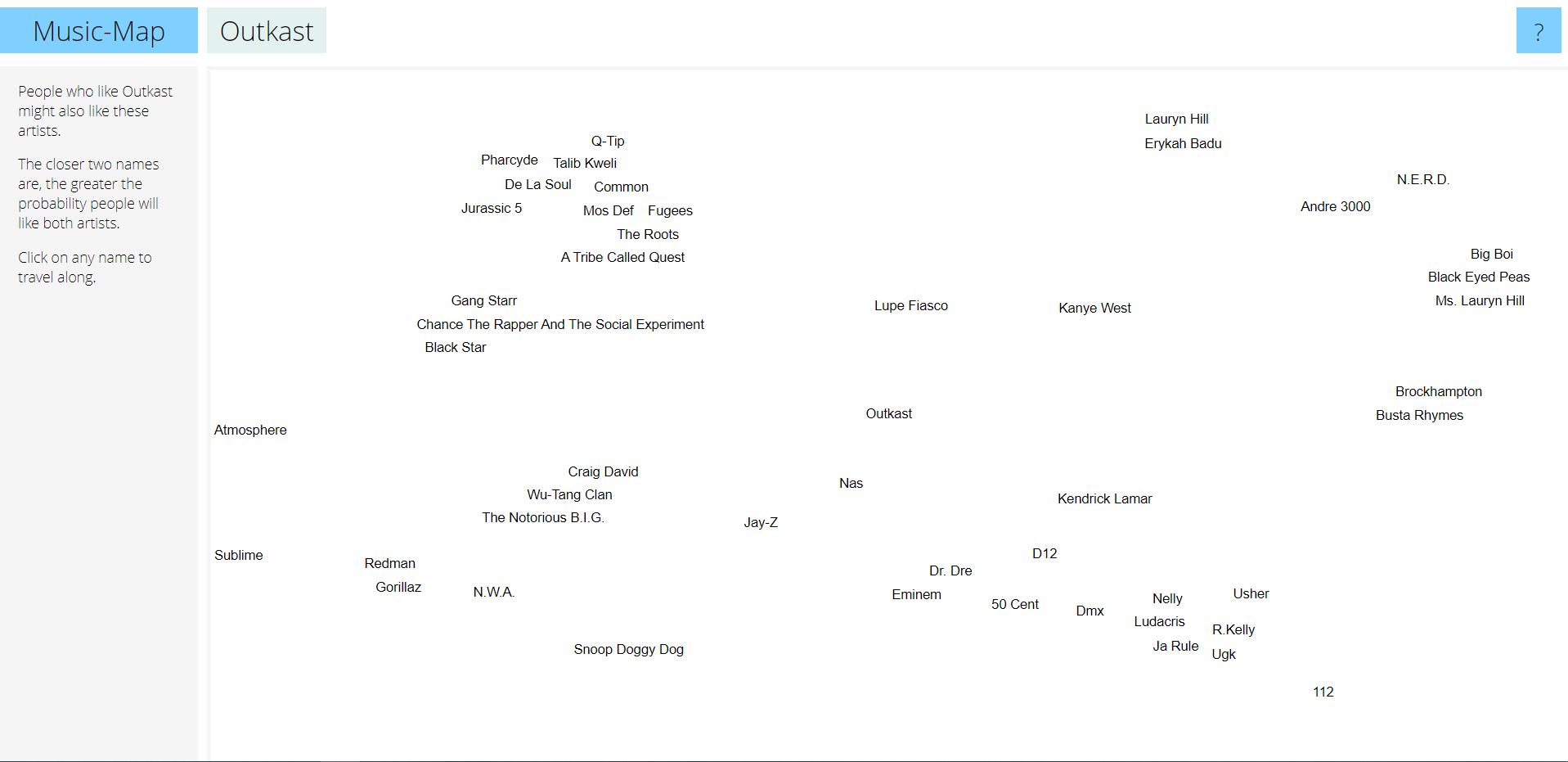
Gnoosic’s Song Map helps you to discover similar music through an interactive map.
While visual maps present a clear picture of your library, song maps connect the dots. Generally, we use maps for navigation; to plot paths from one point to another. This helps us see where things are located in relation to each other. Once you find where you are on a map, you can determine exactly where you’re going and what route to take.
Song maps imitate this process of discovery. A popular service like Gnoosic allows users to enter the name of an artist and discover those that are similar. Whatever you type in will bring up a tree of new artists to look at.
This makes browsing easy, because you already have a clear starting point. The more similar an artist the closer they’ll appear on the map. Type in Eminem and you’ll see Tupac right next to him. Michael Jackson, however, is right at the edge of the map. Interestingly, Eminem has said Tupac influenced his song-writing – song structure is one of the components AI can look at when performing search.
As we’ve seen during the coronavirus quarantine, embracing novelty, whether in technology or content, is both healthy and progressive. AI-powered song maps are useful, intuitive tools to discover new music. If you’re up for adventure, you’ll try what’s on the edges. And if you want something closer to your favourite Jazz musician, you’ve got that too. It even groups the results into clusters. That means if something is a bit different, and you like that, you can find a group of artists that are similarly different.
Similarity Search
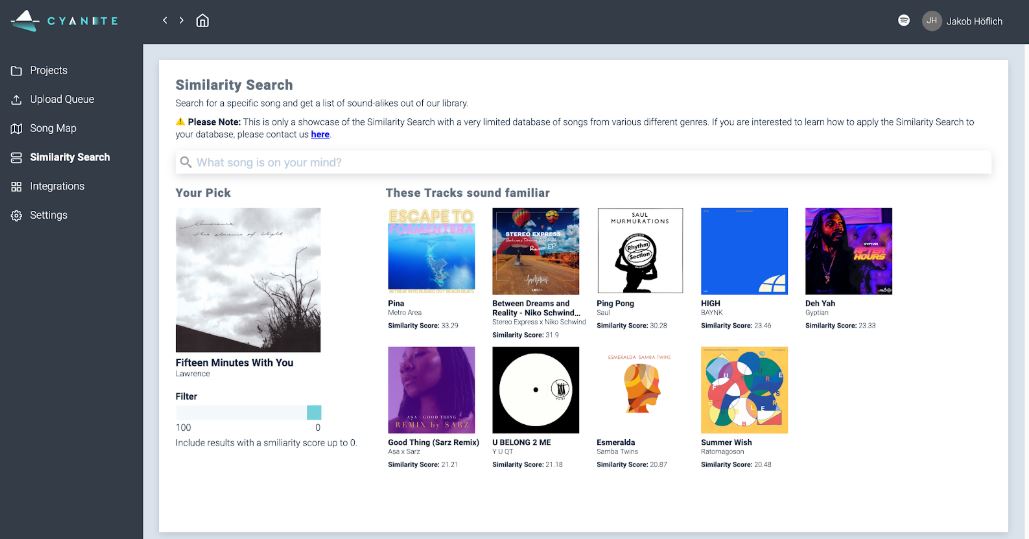
Cyanite’s Similarity Search uses AI to recommend similar songs
Similarity search takes a reference track and gives you a list of songs that match. (This works by pulling metadata and other relevant information from audio files). It does this quickly – we’re talking a matter of seconds.
It’s also more accurate than other methods, because the results are narrowed down to a small selection. Still too many matches? With Cyanite’s Similarity Search, you can filter the AI’s results based on the level of similarity.
This discovery-driven approach emphasises context; the search spans your entire catalogue. This could be helpful to catalogue owners who want to see how different songs within their database relate. You could check if there are more happy than sad songs, for example, and how best to update your library.
It also helps music publishers answer synch briefings faster. They can go from a reference track to the required music quickly, even if they’ve never used the database. You can try out Cyanite’s Similarity Search with a limited database to get a better feel for this application.
With a clear overview of the data, you can prepare your catalogue accordingly. A similarity search approach is functional, specific and visually simple. Users only discover what they’re looking for: the most similar tracks.
If you want better results – whether in your library, catalogue or user experience – delivered by innovative AI technology, give us a shout.
You can schedule a free 15-minute call with our CEO, Markus.
For more of our thoughts on Artificial Intelligence and Music Catalogues, check these out: