Last updated on September 30th, 2024 at 03:12 pm
A couple of weeks ago, Cyanite co-founder Jakob, gave a lecture in a music publishing class at Berlin’s BIMM Institute. The topic was to show and give concrete examples of AI’s real use cases in today’s music industry. The goal was to get away from the overload of buzzwords surrounding the AI topic and shed more light on AI’s actual applications and benefits.
This lecture was well received by the students, so we decided to publish its main points on the Cyanite blog. We hope you enjoy the read!
Introduction
Many people, when they hear about “AI and music”, think of robots creating and composing music. This understandably comes together with a very fearful and critical perception of robots replacing human creators. But music created by algorithms merely represents a fraction of AI applications in the music industry.
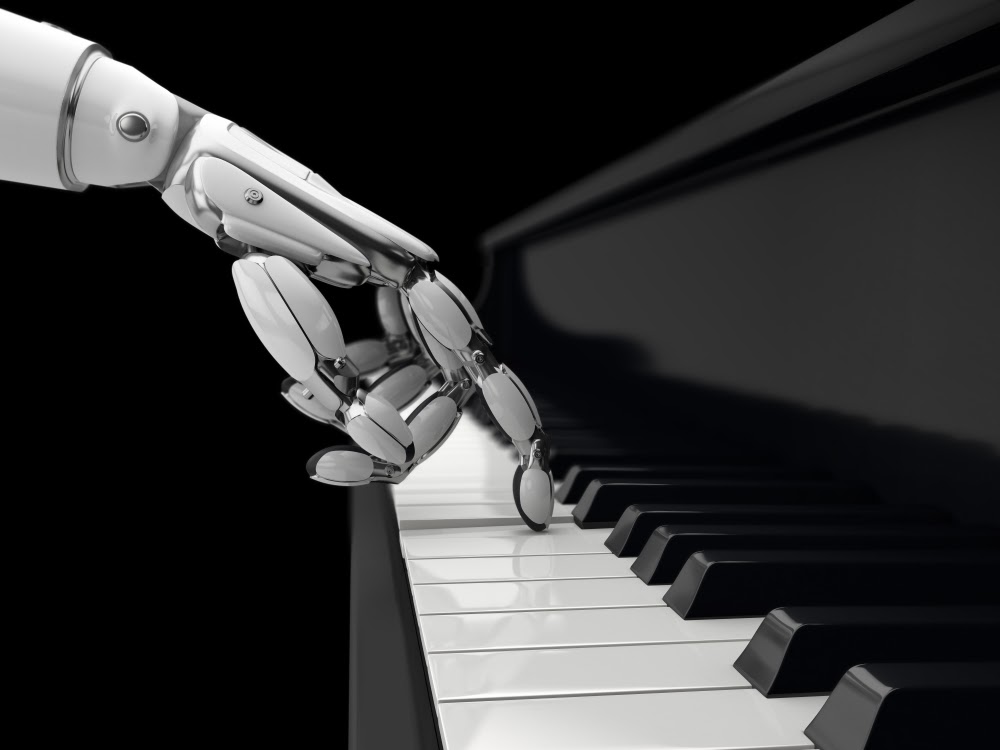
Picture 1. AI Robot Writing Its Own Music
1. Four different kinds of AI in music.
2. Practical applications of AI in the music industry.
3. Problems that AI can solve for music companies.
4. Pros and cons of each AI application.
How does AI work?
Before we dive into the four kinds of AI in the music industry, here are some basic concepts of how AI works. These concepts are not only valuable to understand but they can help come up with new applications of AI in the future.
Just like humans, some AI methods like deep learning need data to learn from. In that regard, AI is like a child. Children absorb and learn to understand the world by trial and error. As a child, you point your finger at a cat and say “dog”. You then get corrected by your parents who say, “No, that’s a cat”. The brain stores all this information about the size, color, looks, and shape of the animal and identifies it as a cat from now on.
AI is designed to follow the same learning principle. The difference is that AI is still not even close to the magical capacity of the human brain. A normal AI neural network has around 1,000 – 10,000 neurons in it, while the human brain contains 86 billion!
This means that AI can currently perform only a limited number of tasks and needs a lot of high-quality data to learn from.
One example of how data is used to train AI to detect objects in pictures is a process called reCAPTCHA. This is a system that asks you to select traffic lights in a picture to “prove you are human”.
The system collects highly valuable training data for neural networks to learn how traffic lights look like.
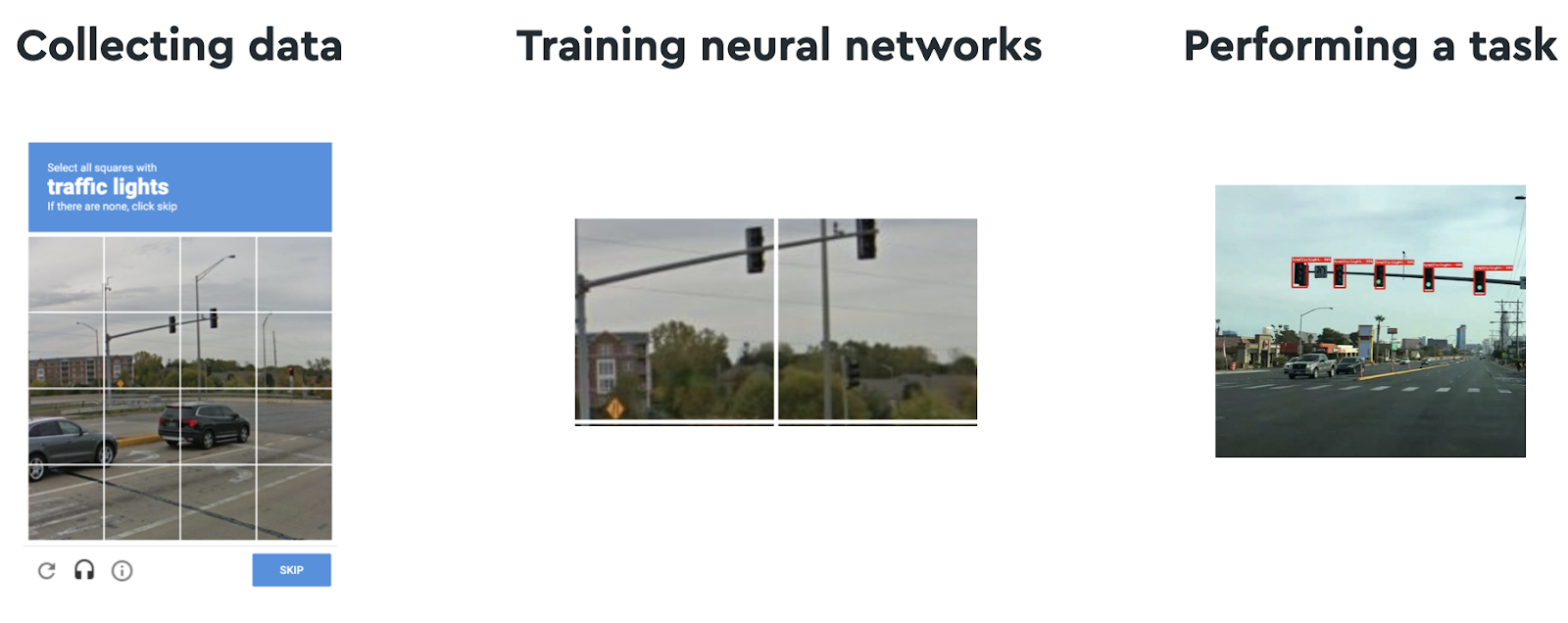
Picture 2. AI Learning with reCAPTCHA
The 4 types of AI in music
Now that you understand the basic AI concept, here is an overview of the four main applications of AI in the music industry. Keep in mind that there are many more possible applications.
1. AI Music Creation
2. Search & Recommendation
3. Auto-tagging
4. AI Mastering
Let’s have a closer look at what problems each area addresses, how the solutions work, and also explore their pros and cons!
Application 1: AI-Generated Music
Problem
Problems that AI can solve in the AI creation field are not very apparent. AI-generated music is, firstly, a creative and artistic field. However, if we look at it from a business context we can identify existing problems. When the music needs to adapt to changing situations, for instance, in video games or other interactive settings, AI-created music can adapt more natively to changing environments.
Solution
AI can be trained to create custom music. For that AI needs input data and then it needs to be taught to make music. Just like a human.
To understand current AI creation capabilities here are a couple of real-world examples:
Yamaha company analyzed many hours of Glenn Gould’s performance to create an AI system that can potentially reproduce the famous pianist’s music style and maybe even create an entirely new Glenn Gould’s piece.
Who is AI-generated music for?
- Game Studios
- Art Galleries
- Brands
- Commercials
- Films
- YouTubers
- Social Media Influencers
Implementation Examples
Pros of this solution
- Cheap to produce new content
- Customizable
- Great potential for creative human & AI collaboration
- Creative tools for artists.
Cons of this solution
- The quality of fully synthesized AI music is still very low
- No concrete application in the traditional music industry
- Legal issues over the copyright including rights to folklore music
- Most AI creation models are trained on western music and can reproduce western sound only
- Very high development cost.
Bottom line
It will take some time for AI-created music to sound adequate or have a straight use case. However, hybrid approaches that use AI to compose music with pre-recorded samples, loops, and one-shots show that the AI-generated future is not far away.
Application 2. Search & Recommendation
Problem
It can be hard to find that one song that fits the moment perfectly, whether it is a movie scene or a podcast. And the more music a catalog contains, the harder it is to efficiently search it. With 500 million songs online and 300,000 new songs uploaded to the internet every day (!!), this can easily be called an inhuman task. Platforms like Spotify develop great recommendation algorithms for seamless and enjoyable listening experiences for music consumers. However, if we look at sync, it gets a lot more difficult. Imagine a music publisher who administers around 50,000 copyrights. Effectively they can oversee maybe 10% of that catalog leaving a lot of potential unused.
Solution
AI can be trained to detect sonic similarities in songs.
Who are Similarity Searches for?
- Music publishers: using reference songs to search their catalog
- Production music libraries and beat platforms
- DSPs that don’t have their own AI team
- Radio apps
- More use cases in A&R (artist and repertoire) and etc.
- DJs needing to hold the energy high after a particularly well-received track (in the post-Covid world)
- Basically, anyone who starts sentences like “That totally sounds like…”
- Managers targeting look-alike audiences.
Implementation Examples
Pros of this solution
- Finding hidden gems in a catalog which goes far beyond the human capacity for search. Here both AI-tagging and AI search & recommendation are employed
- Low entry barrier when working with big catalogs
- Great and intuitive search experiences for non-professional music searchers.
Cons of this solution
- Technical similarity vs. perceived similarity – there is still quite a lot of difference in how a human and AI function. Human perception is highly subjective and may assign higher or lower similarity to two songs, which may be different to what AI thinks.
Bottom line
All positive. Everyone should use Similarity Search algorithms every day.
Application 3. Auto-tagging
Problem
To find and recommend music, you need a well-categorized library to deliver the tracks that exactly correspond to a search request. The artist and the song name are “descriptive metadata”, while genre, mood, energy, tempo, voice, language are “discovery metadata”. More on this topic here. The problem is that tagging music manually is one of the most tedious and subjective tasks in the music industry. You have to listen to a song and then decide the mood it evokes in you. Doing that for one song might be ok, but forget about it at scale. At the same time, tagging requires extreme accuracy and precision. Inconsistent and wrong manual tagging leads to a poor search experience, which results in music that can’t be found and monetized. Imagine tagging the 300,000 new songs uploaded to the internet every day.
Solution
Tagging music is a task that can be done with the help of AI. Just like in the example in the first part of this article, where an algorithm detects traffic lights, neural networks can be trained to learn how, for example, rock music differs from pop or rap music.
Here is a Peggy Gou’s song, analyzed and tagged by Cyanite:
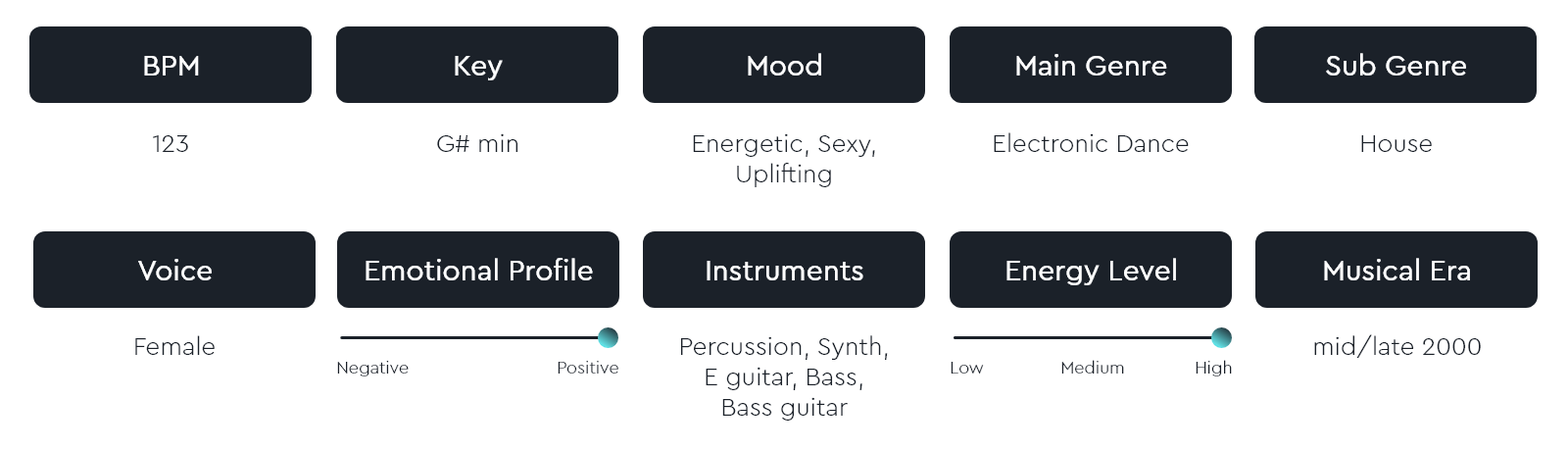
For every music company that knows the pain of manual tagging. If you work in music, chances are pretty high that you had or will have to tag songs. If you pitch a song on Spotify for Artists, you have to tag a song. If you ever made a playlist – you most probably had to deal with its categorization and tagging. If you’re an A&R and present a new artist to your team and say something like, “This is my rap artist’s new party song,” you literally just tagged a song. In all these cases it is good to have an objective AI companion to tag a song for you.
AI-tagging is a really powerful tool at scale. You just bought a new catalog with tons of untagged songs but want to utilize it for sync: AI-tagging is a way to go. You’re a distributor tired of your clients uploading unfinished or false metadata: AI-tagging can help. You’re a production music library that picked up tons of legacy from years of manual tagging: the answer is also AI-tagging.
Implementation Example
In the BPM Supreme library, you can see the different moods, energy levels, voice presence, and energy dynamics neatly tagged by an AI.
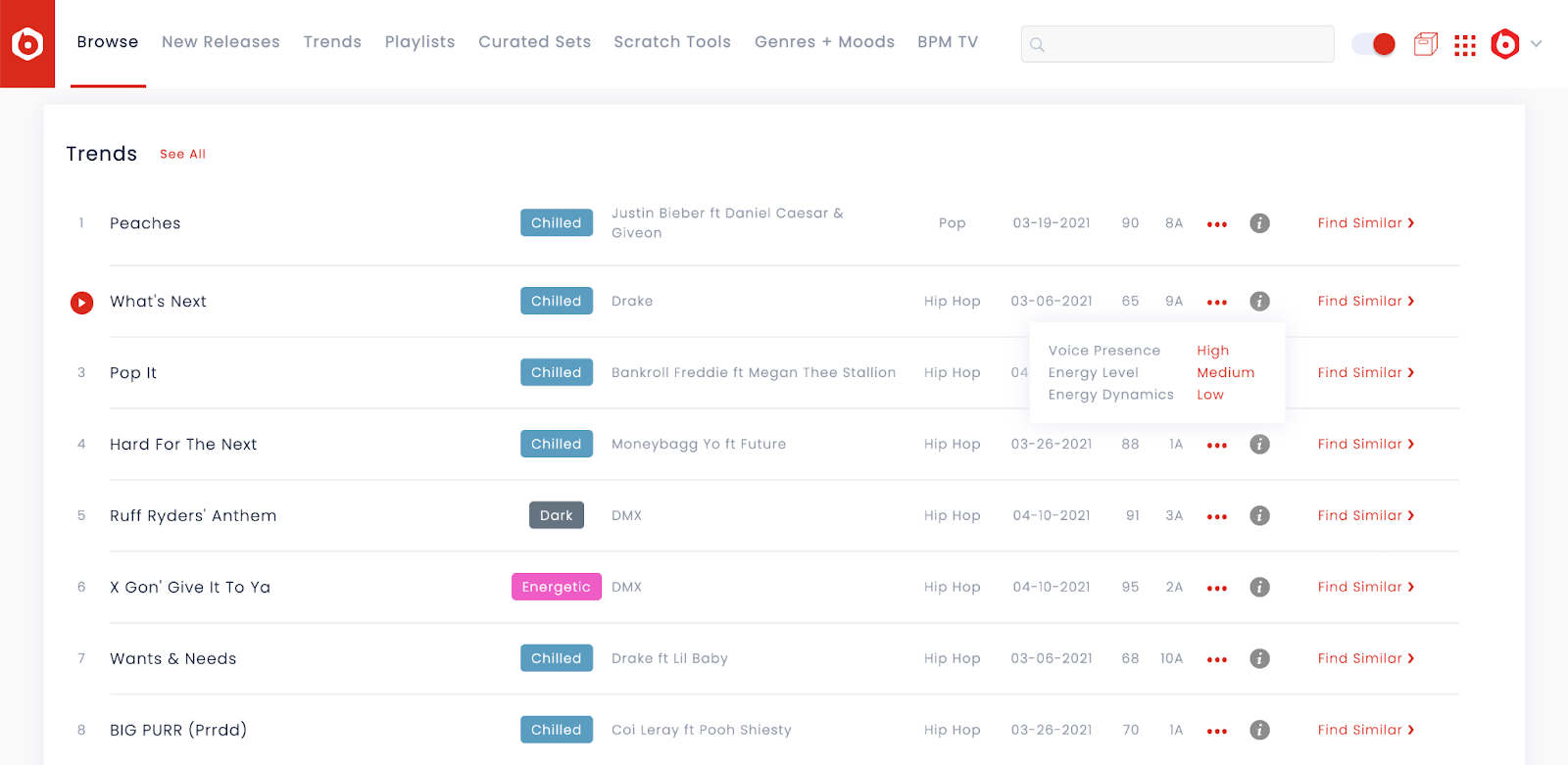
Picture 3. BPM Supreme Cyanite Search Interface
- Speed
- Consistency across catalog
- Objectivity / reproducibility
- Flexibility. Whenever something changes in the music industry, you can re-tag songs with new metadata at a lightning speed.
Cons of this solution
- Development cost and time (luckily, Cyanite has a ready-to-go solution)
- High energy consumption of deep learning models, but still less resource-heavy compared to manual tagging.
Bottom line
Tagging can not replace human work completely. But it’s a powerful and practical tool to dramatically reduce the need for manual tagging. AI-based tagging can increase the searchability of a music catalog with little to no effort.
Application 4. AI Mastering
Problem
Mastering your own music can be very expensive, especially for all DIY and bedroom producers. These categories of musicians often resort to technology to create new music. But in order to distribute music to Spotify or similar platforms, the music needs to meet certain criteria of sound quality.
Solution
AI can be used to turn a mediocre-sounding music file into a great sound. For that, AI is trained on popular mastering techniques and on what humans have learned to recognize as a good sound.
Who is AI mastering for?
- DIY and bedroom producers
- Professional musicians
- Digital distributors
Implementation Example
One company that is leading the field of AI mastering is LANDR. The Canada-based company has a huge community of creators and already mastered 19 million songs. Other players include eMastered and Moises.
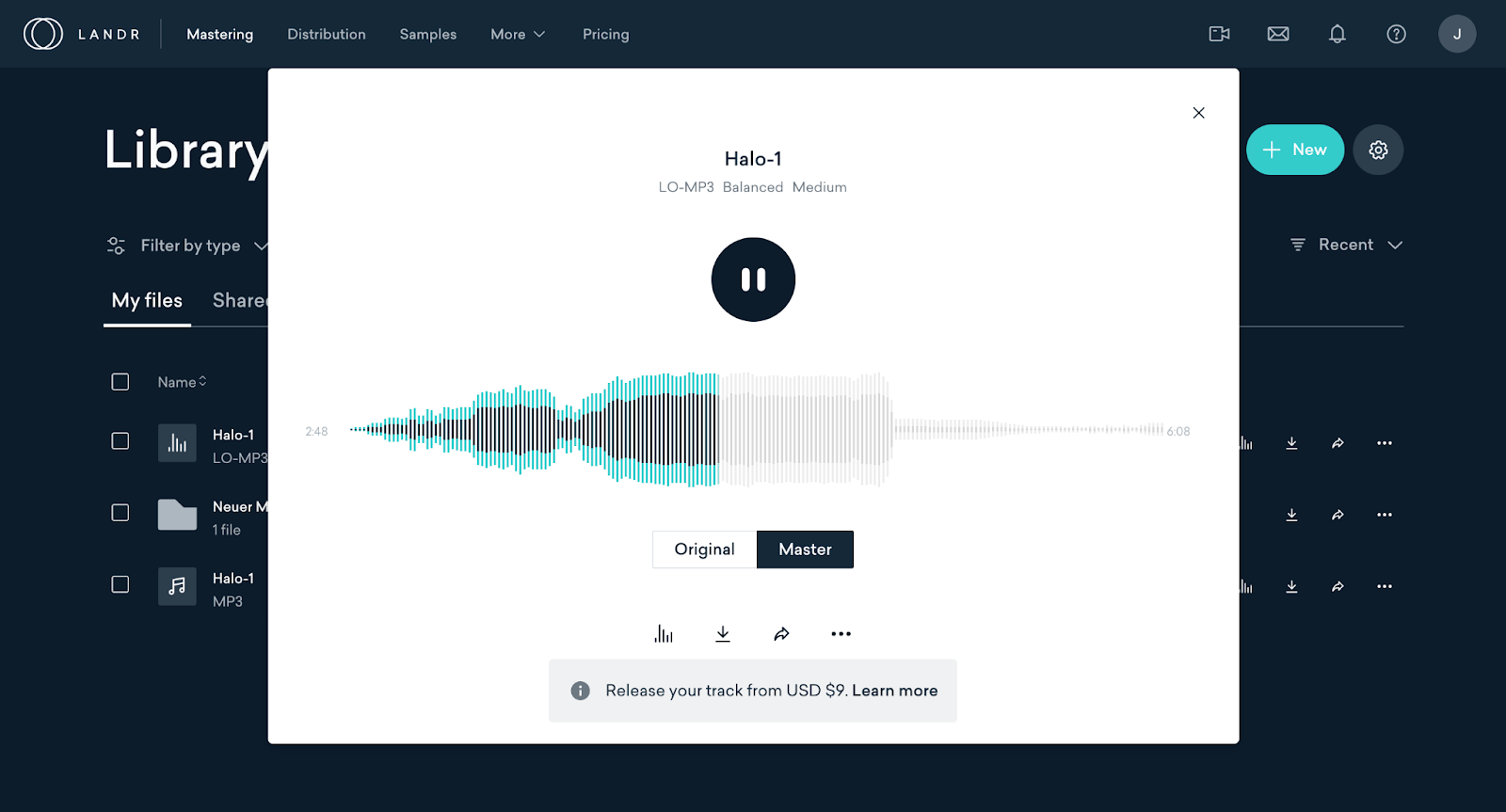
Picture 4. LANDR AI Mastering
- Very affordable ($48/year for unlimited mastering of LO-MP3 files plus $4.99/ track for other formats vs. professional mastering starting at $30/song)
- Fast
- Easy for non-professionals.
Cons of this solution
- A standardized process that doesn’t allow room for experiments and surprises
- Some say AI mastering is “lower quality compared to human mastering”.
Bottom line
AI mastering is an affordable tool for musicians with low budgets. For up-and-coming artists, it’s a great way to get your professionally edited music out to DSPs. For professional songwriters it’s the perfect means to let demos sound reasonably good. Professional mastering experts usually serve a different target group, so these fields are complementing each other rather than AI taking over human jobs.
To sum it up, we presented 4 different concrete use cases for AI, that work for almost every part of the value chain in the music industry. Still, the practical applications and quality differ. AI is far from having the same complex thinking and creativity as a professional music tagger, mastering expert, or musician. But it can already help creatives do their work or even completely take over some of the expensive and tedious tasks.
One of the biggest problems that prevents us from embracing new technology is wrong expectations. There are often two extremes: on the one side, people overestimate and expect more from AI than it can currently deliver e.g. tagging 1M songs without a single mistake or always being spot-on with music recommendations. The other camp has a lot of fear about AI taking over their jobs.
The answer may lie somewhere in between. We can embrace technology and at the same time remain critical and not blindly rely on algorithms, as there are still many facets of the human brain that AI can not imitate.
We hope you enjoyed this read and learned more about the 4 different use cases of AI in music. If you have any feedback, questions, or contributions, you are more than welcome to reach out to jakob@cyanite.ai. You can also contact our content manager Rano if you are interested in collaborations.
For more Cyanite content on AI and music, check these out:
Electronic music experts reveal 4 essential factors on AI-tech adoption for SMEs